Verification Data Analytics with Machine Learning
Verification is data-and computation-intensive, making it an ideal field for ML applications. Advancements in ML have offered many opportunities to accelerate verification workflow, improve verification quality, and automate verification execution. However, being a data-centric method, ML has also elevated data to become the most crucial factor of ML success.
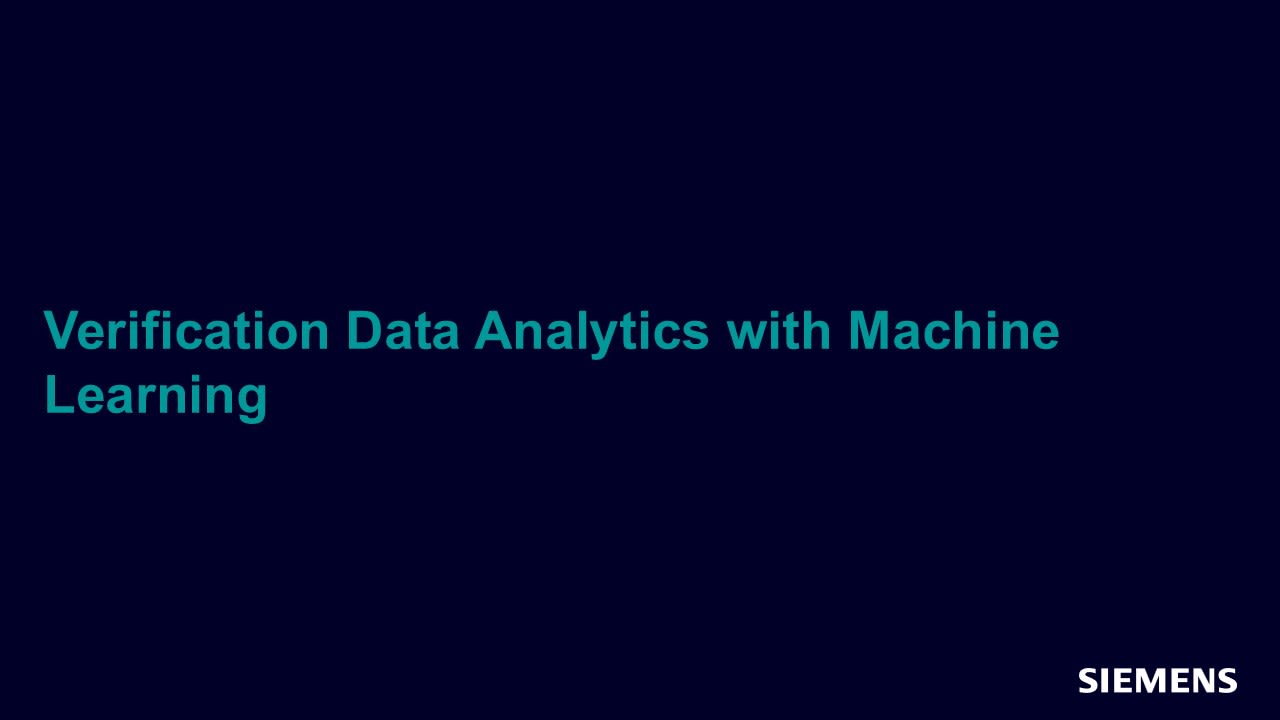
-
Introduction
Ever-increasing design complexity and shortening design-to-market time has demanded faster and more accurate functional verification. Industry surveys indicate that design engineers spend about half of their time on functional verification, and the situation has not improved over the years.
Increasing efforts have been spent on improving verification performance to reverse this trend. With more data gathered from an IC design’s life cycle, it is now possible to gain unprecedented insight by analyzing the data with machine learning (ML).
Recent advances in ML, especially the emergence of large ML models, afford the possibility of gaining knowledge in solving verification problems beyond individual projects or designs. Verification is a data problem, whereas ML is a powerful tool dramatically changing how verification can be done.
ML in a Nutshell
ML development has witnessed exponential growth in the past decades. Plenty of ML techniques have been devised to address challenges in data analytics of every data modality, including numbers, text, audio, image, video, graph, and combinations of multiple modalities. Depending on the data label's availability and the problem's nature, supervised, unsupervised, semi-supervised, or reinforcement learning can be applied to analyze the data. Many ML algorithms were also developed for these data analysis tasks, including regression, instance-based, regularization, decision tree, Bayesian, support vector machine, artificial neural network (ANN), deep learning, dimensionality reduction, and many more emerging algorithms.
Most of the classic tools for verification are rule-based systems. A rule-based system requires domain expert(s) to explicitly develop an algorithm f, which is then coded by the programmer into a usable software tool. The software tool can translate some input data to output data according to predefined rules, as illustrated below in figure 1.
In many cases, such explicit rules are impossible or too complex to obtain, and many quality examples are available. ML becomes a viable solution to solve the problem approximately. These quality examples, called training data, essentially carry enough domain knowledge. A carefully selected ML model can extract the knowledge and represent the algorithm in a function f, which can translate an input to its approximated output, almost as well as the canonical algorithm f. An example of supervised ML is given in figure 1 below.
Figure 1. ML changes the way of solving complex problems from defining and programming rules to collecting and studying data and training ML models
-
Download Paper